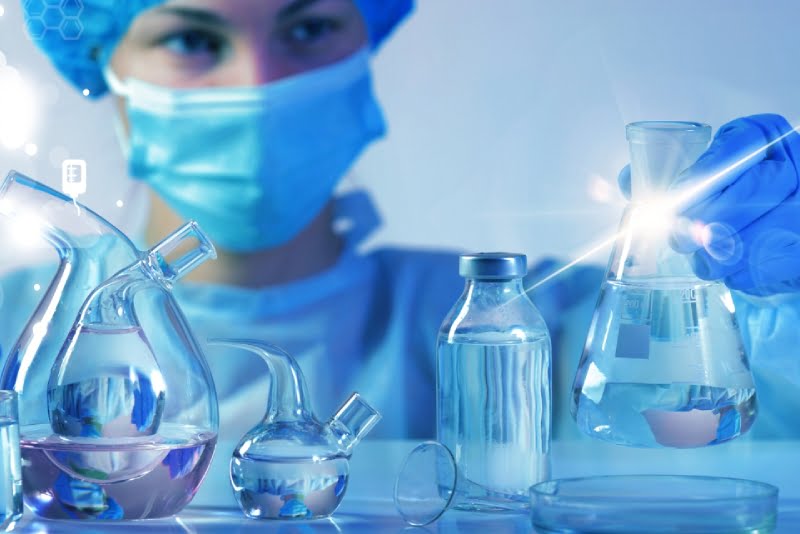
Real-World Data and AI in Pharma: Pioneering Advances in Clinical Trials and Surveillance
May 3, 2024
The integration of Artificial Intelligence (AI) into the pharmaceutical industry represents a significant shift in how clinical trials are designed and how drugs are monitored post-market. This evolution is largely fueled by the utilization of real-world data (RWD) — health-related information derived from various sources outside of traditional clinical research trials. Let's explore how AI is revolutionizing these aspects of pharmaceutical development, promising more efficient, precise, and patient-centric healthcare solutions.
Unpacking Real-World Data: A New Frontier
Real-world data encompasses a broad array of information, including electronic health records (EHRs), insurance claims, wearable technology data, and social media activity. This data reflects the realities of patient behavior and treatment effectiveness in everyday settings, unlike controlled clinical trial environments. The challenge, however, lies in harnessing this extensive, often unstructured, data in a meaningful way.
AI to the Rescue: Transforming Data into Insights
AI, with its ability to process vast amounts of data quickly and accurately, is uniquely positioned to tackle this challenge. Machine learning algorithms can sift through massive datasets to identify patterns and insights that would be impossible for human researchers to find manually. This capability is transforming two critical areas: clinical trial design and post-market drug surveillance.
Enhanced Clinical Trial Design
AI-driven analysis of RWD can dramatically improve the design of clinical trials in several ways:
- Patient Selection: AI can analyze RWD to identify patient populations that are most likely to benefit from a specific treatment. This tailored approach helps in recruiting suitable trial participants, thereby increasing the efficacy and efficiency of trials.
- Predictive Analytics: By predicting potential trial outcomes based on historical data, AI allows researchers to adjust study parameters proactively, reducing costs and time to market.
- Site Optimization: AI tools can identify optimal locations for trial sites by analyzing geographic and demographic data, ensuring higher enrollment rates and more representative sample sizes.
These advancements lead to more robust trial designs that are not only cost-effective but also more likely to succeed in discovering beneficial treatments.
Advanced Post-Market Surveillance
Once a drug is on the market, monitoring its safety and effectiveness becomes paramount. Here, AI integrated with RWD provides powerful tools for ongoing surveillance:
- Signal Detection: AI algorithms can continuously scan data sources for adverse drug reactions, providing early warnings that might not be detected in clinical trials.
- Outcome Prediction: AI can help predict long-term outcomes of treatments in various populations, offering valuable insights into drug performance across diverse patient groups.
- Real-Time Monitoring: Leveraging AI for real-time data analysis enables pharmaceutical companies and regulators to make faster, data-driven decisions about drug safety and regulatory compliance.
Case Studies: AI in Action
Several pharmaceutical giants are already harnessing AI to leverage RWD for better outcomes. For instance, a leading pharma company partnered with tech firms to analyze wearable device data, enhancing their understanding of drug impacts in real-world environments. Another example is a project where AI was used to mine EHRs and claims data to identify unforeseen benefits and risks of cardiovascular drugs, leading to improved patient guidelines.
The Road Ahead: Ethical and Practical Considerations
While the potential of AI in utilizing RWD is immense, it also brings forth ethical and practical challenges. Issues around data privacy, security, and consent are at the forefront, necessitating stringent safeguards and transparent patient communication. Additionally, the accuracy of AI predictions depends heavily on the quality of the data fed into these systems, highlighting the need for standardized data collection and processing protocols.
AI as a Catalyst for Innovation
The symbiosis of AI and real-world data is setting a new paradigm in pharmaceutical research and monitoring. By enabling more precise and efficient clinical trials and enhancing drug safety surveillance, AI is not just streamlining pharmaceutical processes but also paving the way for more personalized and effective healthcare. As we move forward, continuous innovation and collaboration across tech and healthcare sectors will be key to realizing the full potential of this exciting field.
© 2024 ITSoli